Richard Sutton, the father of Reinforcement Learning, will give a lecture at the 49th Prague Computer Science Seminar. Do not miss this once-in-a-lifetime opportunity!
At the Prague Computer Science Seminar on September 14, 2022, we will welcome Richard Sutton from DeepMind and the University of Alberta. He is considered the father of Reinforcement Learning and a true legend in his field. Our center is the proud co-organizers of this event together with the Faculty of Mathematics and Physics at Charles University, where the seminar takes place.
Abstract
Sutton will present a strategic research plan based on the premise that a genuine understanding of intelligence is imminent and—when it is achieved—will be the greatest scientific prize in human history. To contribute to this achievement and share in its glory will require laser-like focus on its essential challenges; identifying those, however provisionally, is the objective of the Alberta Plan for AI research. The overall setting is the familiar one common to many fields (reinforcement learning, psychology, control theory, economics, neuroscience, and operations research): a computationally-limited agent interacts with a vastly more complex environment to maximize reward.
The agent’s machinery is divided into four parts: 1) that which maintains the agent’s situational state (perception), 2) that which maps state to action (policy), 3) that which maps state to expected future reward (value function), and 4) that which maps imagined states and actions to next states (transition model) and enables planning. The Alberta Plan extends this common view to include feature-based subtasks and temporally extended options to solve them; the policy and the value function each become multiple, one each for each of the subtasks and the main task. The setting is then potentially complete and the focus shifts to finding the right abstractions, in state (features) and time (options), and to planning efficiency. The Alberta Plan incorporates continual learning and meta-learning into all of its 12 steps, and expends no effort trying to capture domain knowledge.
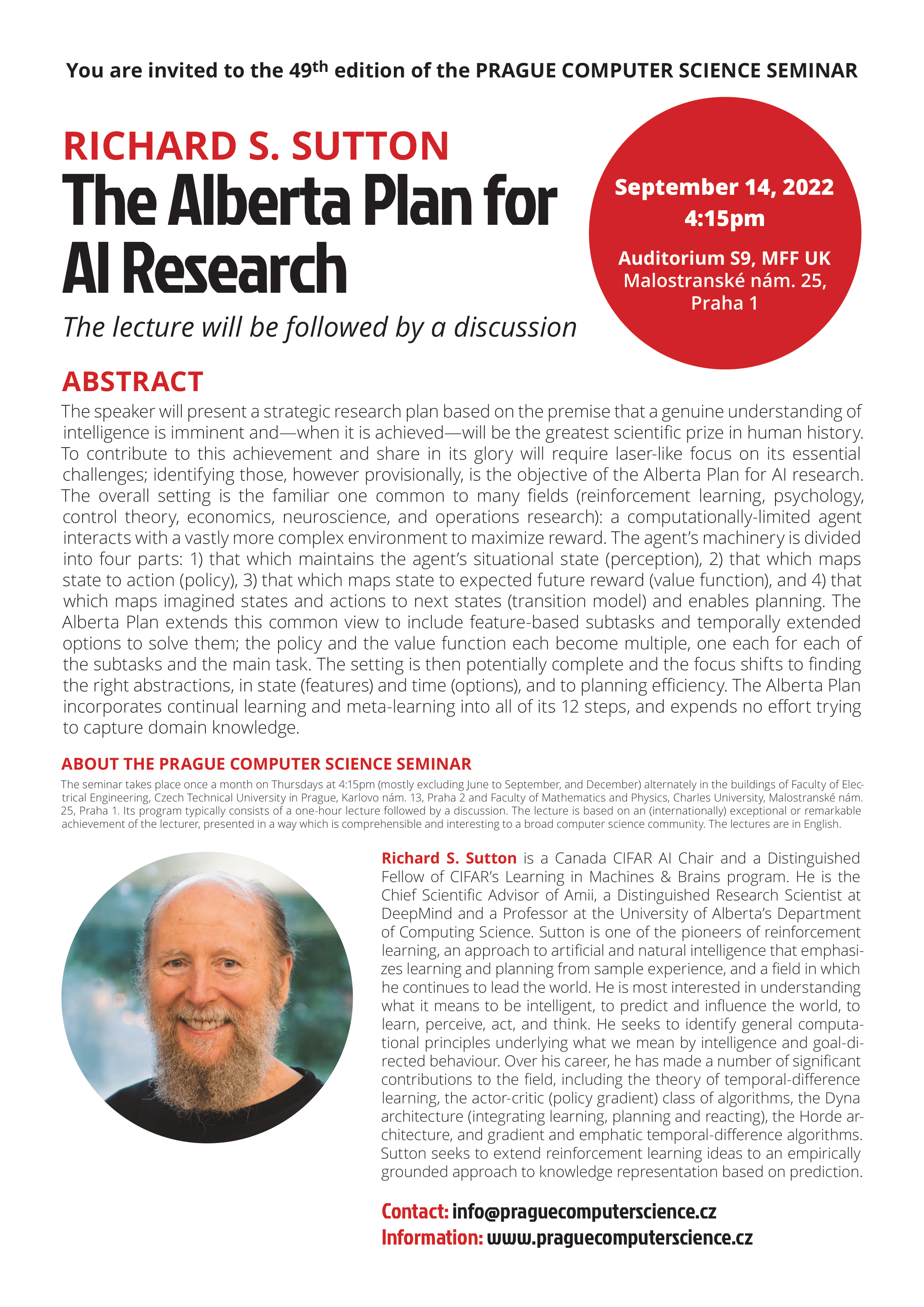
Lecturer: Richard Sutton
Richard S. Sutton is a Canada CIFAR AI Chair and a Distinguished Fellow of CIFAR’s Learning in Machines & Brains program. He is the Chief Scientific Advisor of Amii, a Distinguished Research Scientist at DeepMind and a Professor at the University of Alberta’s Department of Computing Science. Sutton is one of the pioneers of reinforcement learning, an approach to artificial and natural intelligence that emphasizes learning and planning from sample experience, and a field in which he continues to lead the world. He is most interested in understanding what it means to be intelligent, to predict and influence the world, to learn, perceive, act, and think. He seeks to identify general computational principles underlying what we mean by intelligence and goal-directed behaviour. Over his career, he has made a number of significant contributions to the field, including the theory of temporal-difference learning, the actor-critic (policy gradient) class of algorithms, the Dyna architecture (integrating learning, planning and reacting), the Horde architecture, and gradient and emphatic temporal-difference algorithms. Sutton seeks to extend reinforcement learning ideas to an empirically grounded approach to knowledge representation based on prediction.
September 14, 2022 at 4:15 pm
MFF UK (Auditorium 7), Malostranské nám. 25, Praha 1
https://www.praguecomputerscience.cz/?l=en&p=49